Despite being among the most sought after positions in the world of work, there seems to be great confusion about the definitions of Data Scientist, Data Engineer and Data Analyst.
In fact, these are positions that, especially in small and poorly structured companies, often tend to overlap and intertwine.
It goes without saying that the common basis of these roles are represented by the use of the data that are produced and collected in each applicable reality and that are analyzed and used in different phases, with methods and for purposes other than the three figures mentioned.
Clive Humby, British data scientist and mathematician, coined the slogan “Data is the new oil” back in 2006.
In the era of the fourth industrial revolution, data actually represents a strategic resource for economic development. Hence the importance of professionals who are able to “dig” deeply into this amount of information and “extract” their intrinsic value.
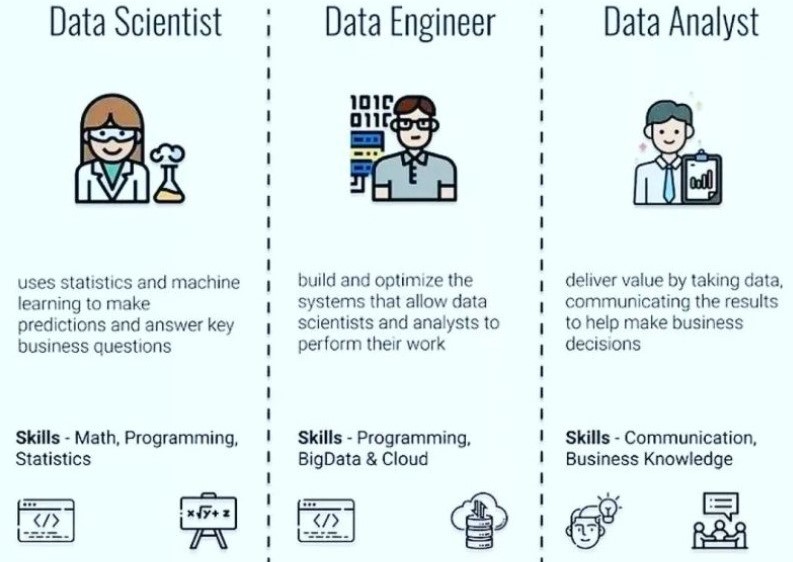
So let’s try to clarify the different denominations of those who work with data:
Data Analyst:
The role of the Data Analyst (also called Business Analyst) can be included among the “users” of the data, or those who answer the business questions relating to the present / past moment (i.e. “How are sales? What has been the trend of the last years … “) through the analysis, polishing and visualization of the collected data.
The main activities of the Data Analyst’s activity are:
- screening and cleaning/polishing of the raw data collected;
- data preparation (data wrangling / munging);
- understanding of business metrics and problems;
- visualization of data through reports and graphs;
- identification of trends and useful suggestions to aid in strategic business decisions.
What are the skills required for this figure?
Surely, a Data Analyst must have sufficient statistical / mathematical / economic training in order to understand the dynamics of said business and interpreting the inherent data.
What tools are used?
It is essential to know Excel and SQL (query language used to manage data in a relational database).
Business Intelligence tools can also be used, such as Tableau, Power BI, QlikView and many others, for creating dashboards, or Python and R to clean/polish and analyze data in greater depth.
Data Scientist:
The role of the Data Scientist also belongs to the ranks of data users. Unlike the Data Analyst, however, the Data Scientist deals with finding solutions regarding the future trend of the business.
Through Machine Learning, Deep Learning techniques and Inferential Modeling, the Data Scientist can find correlations between data and create predictive models on the basis of which he/she can develop recommendation systems useful for said business.
What are the skills required for this figure?
To fill this role it is necessary to have in-depth knowledge of statistics, mathematics and programming. Knowledge of Natural Language Processing (NLP), prescriptive and predicting modeling and multivariate statistics are also very important.
What tools are used?
The Data Scientist also uses SQL, as well as having an advanced knowledge of Python and R. Knowledge of software development and Cloud Services are certainly also useful.
Data Engineer:
The flow of data used by Data Scientists and Data Analysts for their activities is structured by the role of the Data Engineer.
Data Engineers, in fact, build the pipelines from which it is possible to import the information that comes from the users’ devices, thus designing the infrastructure of the data that is collected in the database. Basically, everything that happens to the data before it reaches the database is taken care of by the Data Engineer.
What are the skills required for this figure?
To become a Data Engineer, a thorough knowledge of programming languages and data architecture tools are required. It is also important to have cloud computing and software development skills.
What tools are used?
This figure uses SQL related tools, such as MySQL and PostgreSQL; he/she also should know technologies related to non-relational databases (NoSQL), such as MongoDB and Cassandra; and they use data tools such as Hive, Spark, Kafka, Pig and Map Reduce; As for cloud technologies, tools such as AWS, Google Cloud and Microsoft Azure are used.
From this brief analysis, the wide overlap of the three figures clearly emerges in terms of skills and tools, which are anything but exclusive and constantly evolving.
Author: Claudia Paniconi | Head of Marketing DMBI Photo by Campaign Creators on Unsplash